Digital Platform and Artificial Intelligence Futures
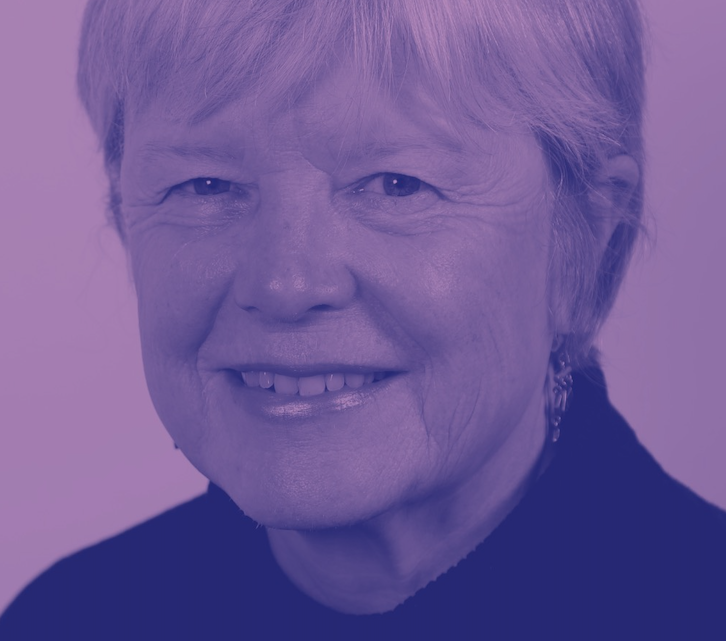
Central to the development of a digitally enabled world are innovations in technologies variously labelled Artificial Intelligence (AI), predictive algorithms or big data analytics. AI technologies rely on practices extending beyond passive capture of data to include methods of deepening and intensifying user engagement with digital platforms. In the 1950s, the AI aspiration was to discover whether characteristics of human intelligence such as learning, problem solving and heuristic formation could be convincingly simulated using computer hardware and software. With a few exceptions, expectations for what is known as ‘general AI’ had singularly unimpressive outcomes. Some researchers remain sympathetic with the aim of achieving the reproduction of human cognitive and rational capacities or “intelligence”, i.e., applications including adaptive learning, sensory interaction, reasoned planning and creativity.
With very large data sets, ‘narrow AI’ currently aims to use data to create systems that can reproduce or mimic the observed behaviour embodied in a dataset. Traditionally, algorithms were rules-based with finite, deterministic and effective collections of steps for transforming inputs into outputs. In contrast, today’s data-driven approach to AI involves discovering the rules of the algorithm from the data. Innovations in data-driven AI techniques are being employed by today’s digital platforms in multiple contexts from search to news aggregation, monitoring, forecasting, filtering and scoring. However, these data-driven approaches lack the systematic rule-checking available in rules-based algorithms. When an AI system has been created from data it is generally not clear whether it would produce different results if other, equally or more valid, datasets were used. The outputs or answers it produces may exceed or fall short of human capabilities.
The platform companies are large-scale investors in the science underlying AI and its practical application, and they are benefitting from the claimed predictive value of AI techniques. They are also benefitting from the availability of “big data” because this resource flows freely to them as the result of the governance rules which are currently in operation. This applies both to user-generated data and to multiple “open data” repositories. This has given the platform operators an important first-mover advantage in developing AI applications and it reinforces other sources of their market power which stems from economies of scale resulting from the acquisition of data.
All these developments have implications for trust in AI applications. Whether AI predictions can be trusted hinges upon whether humans grant deciding power to AI-empowered systems. When AI systems are granted the main or sole responsibility to make decisions, assurance is needed that these systems are reliable and accountable and that they do not bypass human rights to transparent processes and non-discrimination. Decisions based on the output of data-driven algorithms classify individuals. When decisions are taken based on these classifications, they affect people’s life chances. If uncertainty is high, there are consequences for decision making. The typical aim of the use of prediction engines is to produce reliable and fair results, “without bias”, but bias arises from, and is inherent within, any social structure. Bias is reflected in data that is used in constructing data-driven AI systems and in human supervision of these systems. Those who must interpret results have little or no capacity to correct for biases in order to avoid incorrect inferences.
Uncertainty associated with these systems raises concern about the use of data-driven AI systems to underpin decisions about access to public services, interpretations of surveillance data and the customisation of online services. Despite their claimed “reliability” in some of these contexts, regularity of prediction provided by AI systems is not the same as a claim to reliable outcomes from the point of view of equity or fairness. As learning machines and algorithms extend their reach throughout society, new approaches to their governance and to ethics may alleviate some of the potential problems. But if citizens’ fundamental rights are to be respected, analysis of the AI applications will need to move beyond a narrow framing of issues to address equity and inclusion and human autonomy. What the socio-political and economic consequences of AI and the digital platforms’ use and supply of these systems are for societies depends upon whether applications are, on balance for better or worse, and on contests over their use.
These and other issues related to the increasing dependence of societies on digital platform initiated commercial datafication strategies are discussed in Mansell, R. and Steinmueller, W. E. Advanced Introduction to Platform Economics. Edward Elgar Publishing, 2020; chapter 1 is at http://eprints.lse.ac.uk/106205/