Prediction of misleading news stories with an F1-Score of 98%
By KatalinFeherFulbrighter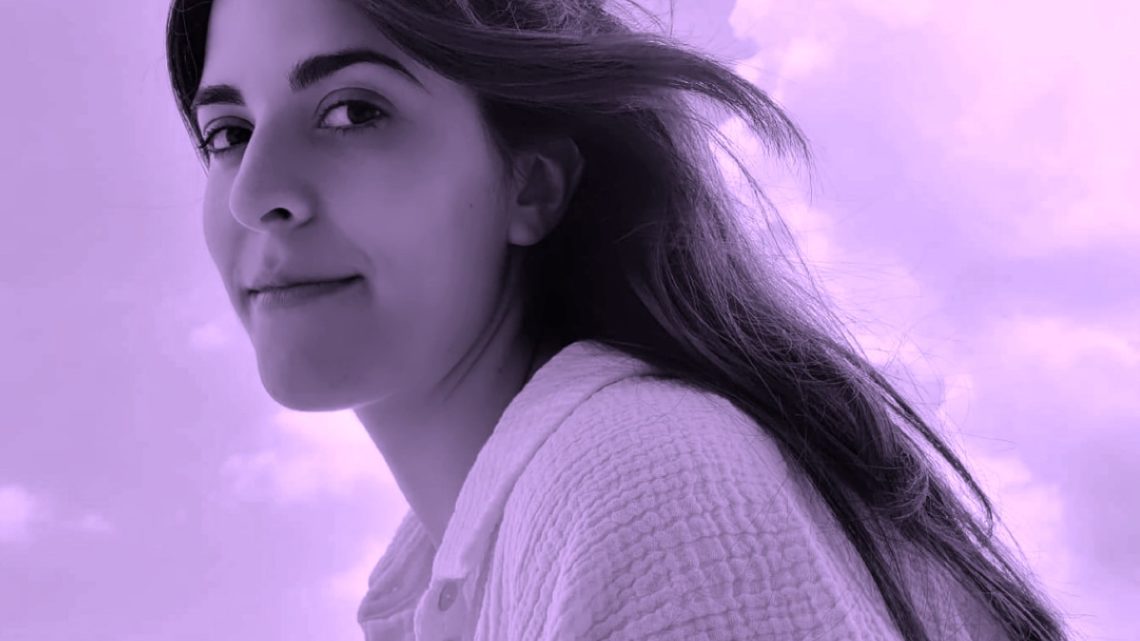
The debate about fake news is more relevant than ever. The diffusion of untruthful content can intentionally serve purposes such as financial and political interests, affecting society and consequently democracy. Recent important events, such as the Covid-19 pandemic and the war in Ukraine, highlight the significance of news verification, the growing need for fact-checking, and the challenges that journalists face as they have to manually and efficiently check myriad requests of potential deceptive information daily. There is a clear need for an immediate way of tracking falsehoods before they become widely spread. Hence, the rise of fake news has attracted strong interest from computer scientists and media researchers who embrace the age of AI by employing machine learning and other automated methods to help identify disinformation.
Our research proposes a computational approach to detect potentially fake information by identifying textual and non-textual attributes of both fake and real news articles and then using explainable machine learning methods for disinformation prediction. The proposed model in addition to content-based features related to language use and emotions, harnesses the predictive power of users’ interactions on the Facebook platform, and forecasts deceptive content in (i) news articles and in (ii) Facebook news-related posts. More precisely, the content-based features that we examined were linguistic, such as body length, title length, uppercase letters, parts of speech, noun-to-verb ratio, lexical diversity, readability, abusive vocabulary, title and body similarity, subjectivity, and also emotional features such as the four basic emotions, anger, fear, joy, sadness and the overall affect that includes the level of valence, arousal, and dominance. The engagement features were about the reaction of “like”, “love”, “wow”, “haha”, “sad”, “angry”, the total number of interactions, the number of shares and comments, and the overperforming which is based on the performance of similar posts from the same page in similar timeframes.
For this study, we gathered news content from both trustworthy and unreliable English-language websites by creating a dataset consisting of a total of 23.420 articles, and then we searched for analytics on each article in our dataset posted on Facebook resulting in a second smaller dataset of a total of 9.644 articles. For the data analysis, we separated the experiment into two phases based on the two different datasets. In Phase A, the whole dataset of the fake and real articles was used for the evaluation of the importance of only the content-based features, notably the linguistic and emotional features. Then, in Phase B, so as to highlight the predictive power of the engagement features, the second dataset, which except for the articles also included the engagement features, was used twice, once using only the engagement features as predictor variables, and the second time combining all the features. For the analysis, we opted tree-based models, namely the Random Forest classifier and Decision Tree classifier, which provide in-depth explanations of the predictions.
The findings of the study show that the algorithm with the highest accuracy was Random Forest, which is able to predict misleading news stories with an F1-Score of 98% based on content-based features, notable capitals in the main body, headline length, the total amount of nouns and numbers, lexical diversity, the emotion of arousal, and the engagement feature of Facebook “likes”.
The paper provides valuable insights concerning the fake news attributes useful in the light of combating disinformation, and a machine learning approach is proposed to automatically detect false stories and point to certain telling characteristics of these falsehoods. Therefore, this research on spotting patterns in digital news stories is an AI-driven solution that can mitigate the problem of spreading fake news and also support the work of fact-checkers. The approach can potentially be incorporated into media literacy education programs to bolster resilience against this devastating phenomenon.
Based on the particular research, future work could combine a humans-in-the-loop approach by asking actual fact-checkers to define potential deceptive stories based on certain identifiers and their respective significance and then correlate their judgment with the feature importance of the model.
_____
SOURCE: ”Evaluating the Role of News Content and Social Media Interactions for Fake News Detection”, which was published in proceedings of the Third Multidisciplinary International Symposium on Disinformation in Open Online Media, MISDOOM 2021, held in September 2021 https://doi.org/10.1007/978-3-030-87031-7_9
Our proposal for improving the credibility and robustness of information can be found here: You are welcome to contact me by email: anakaram@media.uoa.gr
Anastasia Karampela is a researcher at the Laboratory of New Technologies in Communication, Education, and Media of the National and Kapodistrian University of Athens. Her research interests are related to the integration of data science and ΑΙ technologies in the media field, more specifically AI for fake news detection, natural language processing, and data journalism.